Generative AI vs Agentic AI
Generative AI vs Agentic AI
Blog Article
Introduction
Artificial intelligence’s rapid integration has revolutionized how companies manage information, safeguard it, and make decisions. However, not all AI systems are made equal. Two new AI paradigms are changing automation, intelligence, and interaction: generative AI and agentic AI. Understanding these distinctions is essential for organizations handling sensitive data to use AI responsibly for governance, privacy, security, and business agility.
What is Generative AI?
Based on user input, generative AI systems produce novel content. Depending on the input, they can produce text, photos, music, video, or code using deep learning models and large datasets. Important characteristics include:
–Reactive nature: Rather than acting on their initiative, these systems react to human input.
–Focus on content creation: Their main duty is to provide fresh material that imitates previously learned patterns.
–Pattern recognition: They are very good at finding and reproducing patterns in large amounts of training data.
–User-guided operation: The output’s context and tone are determined by human input.
Microsoft Copilot and OpenAI’s ChatGPT are well-known examples that have revolutionized software development, design, content marketing, and other fields.
What is Agentic AI?
An advanced development in AI is agentic AI. These systems do more than simply create information; they also carry out tasks, make choices on their own, and manage intricate workflows. Among the fundamental traits are:
–Proactive approach: Without continual human input, agentic systems take action to achieve objectives.
–Autonomous operation: Based on real-time input, they continually modify their strategy.
–Contextual adaptation: They assess how the environment is changing and adjust their strategy to suit changing demands.
–Capabilities for making decisions: They assess choices and choose the optimal course of action using reasoning engines.
While generative AI creates material to answer particular questions, agentic AI manages more general processes on its own, which makes it perfect for multi-step job execution and ongoing adaptation.
How does agentic AI work?
Large language models may now make decisions on their own thanks to agentic AI. Features of its framework:
–Perception mechanisms: Gather information from a variety of sources, including social media, databases, and Internet of Things devices.
–Reasoning engines: Use sophisticated reasoning to plan multi-step tasks.
–Action execution: Use external tools and APIs to do our tasks.
–Learning systems: Constantly updating their database in response to results and feedback.
By combining observation, reasoning, action, and ongoing learning, agentic AI can manage intricate workflows and adjust to novel situations much like a human team member.
Applications of generative AI and agentic AI
Despite their very diverse functionalities, both generative and agentic AI are utilized in a variety of industries.
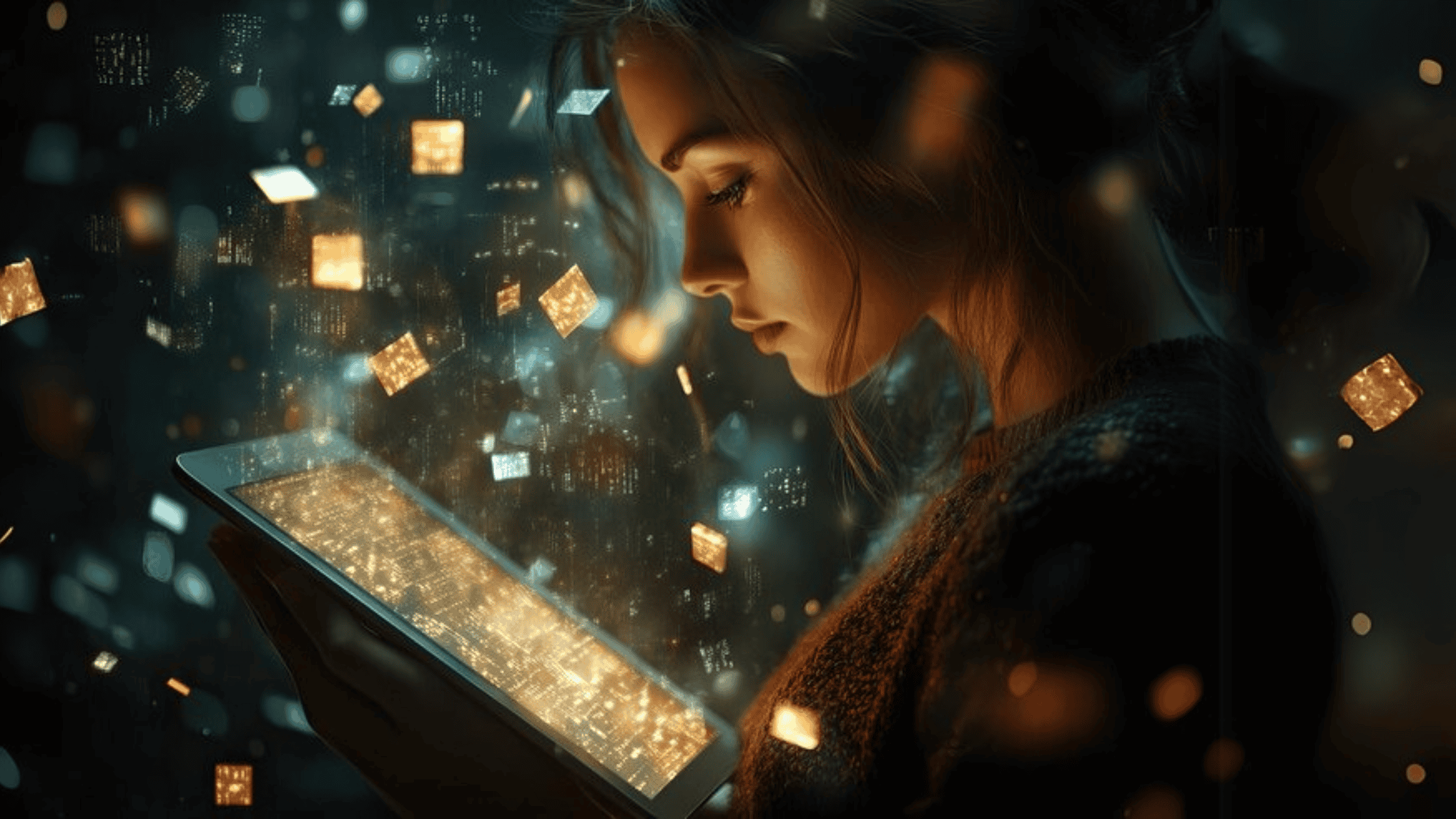
Generative AI finds applications where content generation is essential. Typical usage scenarios include of:
To reduce time and ensure consistency, content marketing involves automating the creation of articles, social media postings, and marketing copy.
–Descriptions of products: Creating original descriptions that draw readers in and raise search engine results.
–Data analysis: Finding patterns and insights in big datasets is known as data analysis.
–Software development: To speed up development cycles, help with code production, documentation, and debugging.
–Design support: Producing visual information from written descriptions to aid in the work of creative teams.
Agentic AI, which can make decisions on its own, is better suited to more complex applications. Important use cases include:
–SEO management: This includes keyword research and optimization, backlink analysis, and real-time strategy adjustments.
–Customer service: Managing channels of help and addressing problems with little assistance from humans.
–Technical improvements: These include controlling schema markup, mobile friendliness, and website performance.
–Workflow automation: managing resource allocation and coordinating intricate operations across platforms.
–Tracking performance: Monitoring measurements and producing insights to improve operations is known as performance tracking.
Agentic AI provides a major boost in operational efficiency by driving both routine operations and strategic decision-making.
Limitations of Generative AI and Agentic AI
–Risks to Data Privacy: Generated material may inadvertently contain sensitive information.
–Bias and Misinformation: Using faulty training data, AI may provide biased or inaccurate narratives.
–Intellectual Property Issues: Copyright and ownership concerns are brought up by AI-generated material.
Challenges of Agentic AI
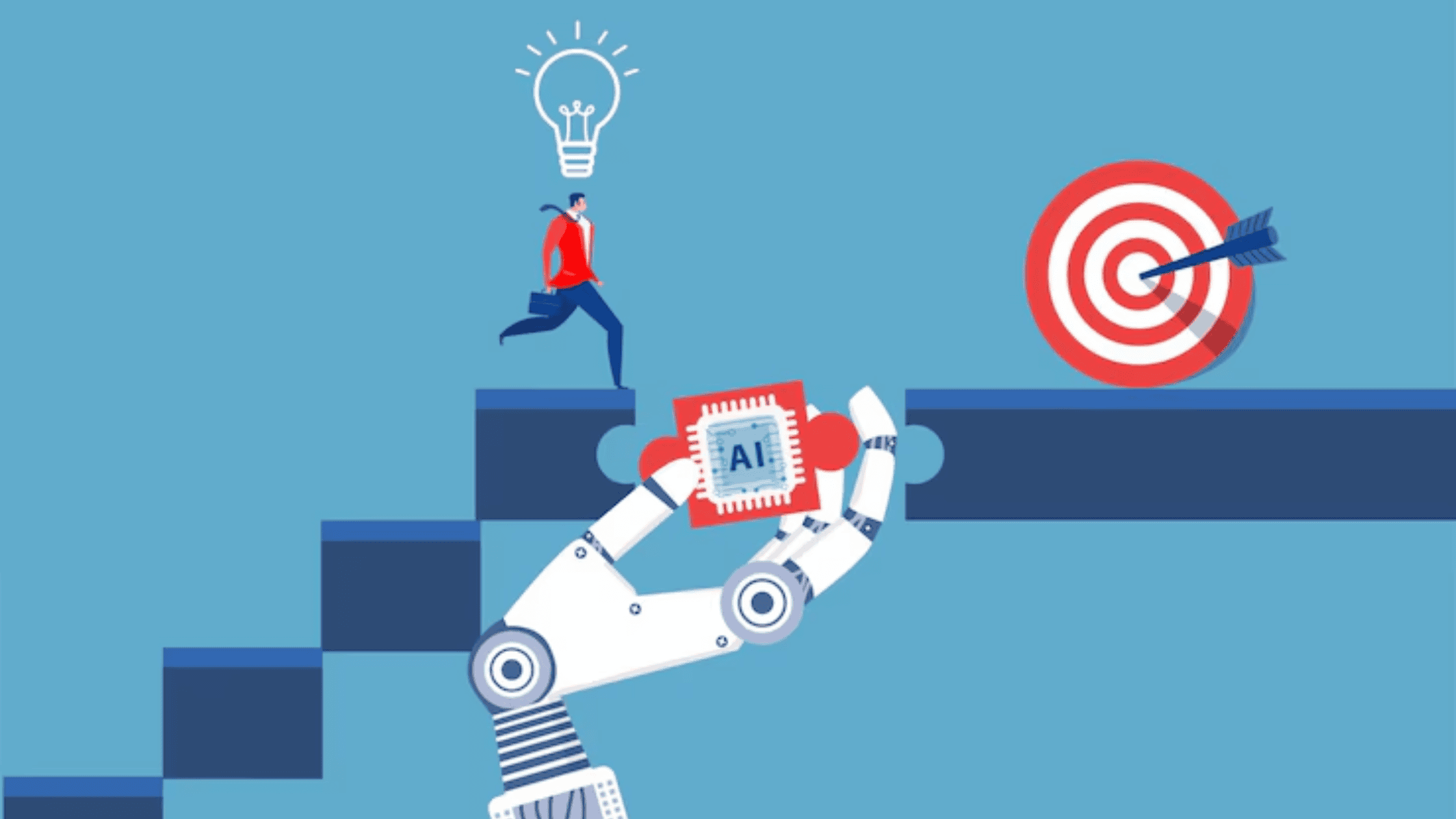
–Control and predictability: Autonomous decision-making can be challenging to monitor.
–Ethical Concerns: Decisions made without human oversight may pose risks.
–Security Concerns: If hacked, autonomous AI might create unforeseen disruptions.
Future of AI
The distinction between generative and agentic AI is predicted to fade in the future as hybrid systems emerge that combine creative output with autonomous decision-making capabilities. Expect the following advancements:
Hybrid systems that perform tasks autonomously while dynamically creating personalized material.
Collaborative frameworks for improving human-AI interactions, with AI managing background execution and decision-making.
Adaptive learning enhances performance over time in response to real-world input.
Enhanced autonomy, where the distinction between automated execution and content production is blurred.
To prepare for these improvements, businesses should:
-Develop AI literacy to better understand AI’s capabilities and limitations.
-Create adaptable, cloud-native infrastructures that can accommodate changing AI models.
-Encourage a culture of creativity by organizing pilot projects, hackathons, and continual learning.
-Establish ethical guidelines for data privacy, fairness, and transparency.
Final Thoughts
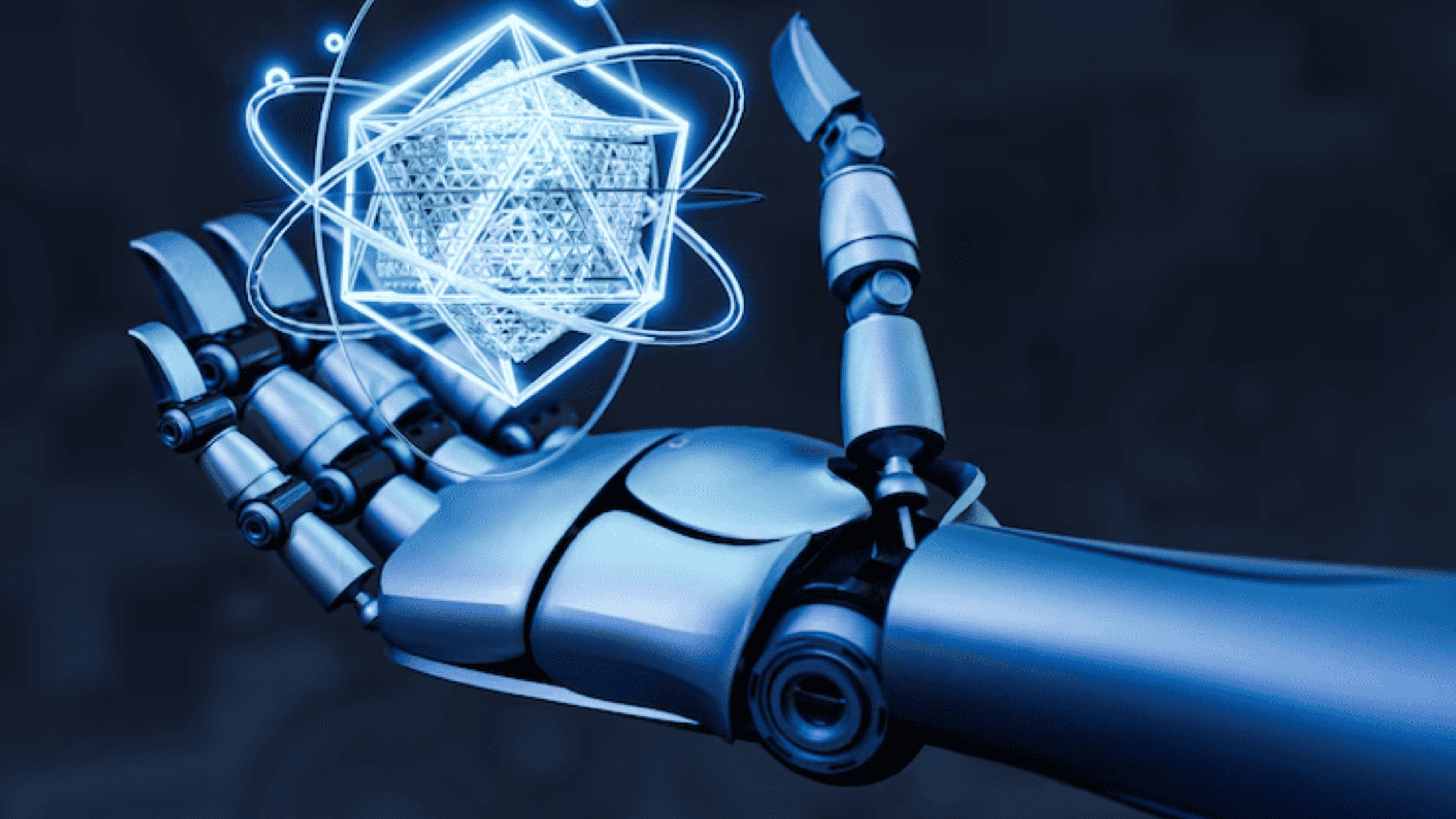
AI technology is progressing toward a future in which the line between content production and autonomous decision-making blurs. Generative AI development companies have revolutionized creative industries such as content marketing, design, and software development. Meanwhile, agentic AI is poised to transform operational workflows with completely autonomous systems that carry out complicated tasks with minimal oversight.
In today’s cloud-native world, novel platforms are constantly emerging. Alignminds, AI Development company in US, for example, is attempting to integrate AI across the software development lifecycle, allowing non-experts to create intelligent apps with natural language prompts and AI advice. As businesses attempt to decrease costs and improve operations, adaptive, agent-first solutions are becoming increasingly important. Report this page